As companies rush to adopt generative AI, many overlook a critical technology that can determine the success of their AI initiatives: vector databases. Understanding and implementing vector databases is not just a technical consideration—it’s a strategic necessity to distinguish successful AI adopters from those who struggle to keep pace.
The pressing need for vector databases
Gartner forecasts that by 2026, over 70% of generative AI applications will rely on vector databases. This represents a fundamental shift in how businesses will manage and use their data for AI. Companies that act now are already seeing significant advantages over their competitors.
The urgency stems from the increasing complexity of data that generative AI models must process. These models work with vast amounts of unstructured information – text, images, audio, and video. Traditional databases struggle with this type of data, while vector databases are designed to handle it efficiently.
AI systems, as they become more sophisticated, require faster data retrieval to maintain real-time performance. Vector databases offer superior speed for similarity searches and scale more effectively as data volumes grow. This improved speed and scalability directly translates to better user experiences and more efficient operations.
Vector databases also enable more nuanced and context-aware searches, leading to more accurate AI outputs. This increased accuracy means better customer experiences and more reliable insights for businesses. While implementation requires an initial investment, vector databases can significantly reduce long-term computational costs by optimizing data storage and retrieval.
Businesses that delay adopting vector databases risk falling behind in their AI capabilities. Having the right data infrastructure in place will be crucial for capitalizing on AI’s potential.
Why company leaders need to pay attention
Vector databases are a strategic asset that can drive significant business outcomes. Here’s how they translate into tangible benefits that directly impact your bottom line.
Market Positioning and Competitive Advantage
By enabling faster, more accurate AI responses, vector databases allow you to outpace competitors in product development and customer service. For instance, e-commerce companies using vector databases can offer more precise product recommendations, potentially increasing conversion significantly. In financial services, faster data processing can lead to split-second trading decisions, potentially increasing returns by several percentage points.
Revenue Growth
The ability to extract value from unstructured data opens new revenue streams. Media companies can monetize their content more effectively by offering highly personalized experiences, potentially increasing subscriber retention by 25%. Healthcare providers can analyze medical images and records more efficiently, leading to faster diagnoses and improved patient outcomes, which can increase billable services and patient satisfaction scores.
Cost Savings and Operational Efficiency
Vector databases optimize data processing, significantly reducing the computational costs of running large AI models. This can lead to a 40-60% reduction in cloud computing expenses for AI operations. Moreover, the scalability of vector databases means you can grow your AI capabilities without proportional increases in infrastructure costs, improving your long-term cost structure.
Risk Mitigation and Compliance
In heavily regulated industries like finance and healthcare, vector databases enhance fraud detection and compliance monitoring. By processing vast amounts of transaction data in real time, financial institutions can potentially reduce fraud losses by up to 60%. This not only saves money but also protects your brand reputation.
Innovation Catalyst
Vector databases enable you to process and analyze data types that were previously challenging to work with, such as audio, video, and complex text. This can spark innovation across your organization. For example, manufacturers can use AI to analyze sensor data from production lines, potentially reducing defects by 50% and significantly improving product quality.
Customer Experience and Loyalty
With vector databases, you can create hyper-personalized customer experiences at scale. Retail companies have seen increases in customer lifetime value of up to 20% by offering more relevant product recommendations and personalized marketing. In the service industry, more accurate chatbots and virtual assistants can resolve customer queries faster, potentially reducing call center volume by 35% and significantly improving customer satisfaction scores.
Talent Attraction and Retention
Being at the forefront of AI technology makes your company more attractive to top talent. Engineers and data scientists are drawn to organizations using cutting-edge technologies like vector databases, potentially reducing recruitment costs and time-to-hire for critical roles by up to 25%.
By implementing vector databases as part of your AI strategy, you’re not just adopting a new technology—you’re positioning your company for sustained growth, increased efficiency, and a strong competitive advantage in an AI-driven business landscape.
Action steps for decision-makers
Let’s take a look at some actionable steps decision-makers can make to evaluate and implement vector databases.
1. Evaluate Your Data Systems: Start by assessing your current data infrastructure. Determine if your existing systems can handle the volume, variety, and velocity of data required for generative AI. Evaluate whether they can support the complex data processing demands that vector databases require, mainly for handling unstructured data like text, images, and audio.
2. Conduct a Proof of Concept: Test vector database integration on a small scale before a full rollout. Start with specific projects, such as improving search capabilities or delivering personalized customer recommendations. This approach allows you to measure the performance improvements and understand any technical adjustments needed before scaling up.
3. Develop Clear Evaluation Metrics: Establish key performance indicators (KPIs) to measure the success of your vector database implementation. These metrics could include query response time, data retrieval accuracy, user experience improvements, cost savings in computational expenses, and the impact on specific business outcomes, such as increased customer satisfaction or reduced operational costs.
4. Train Your Team: Invest in upskilling your data scientists and engineers on vector database technologies. They should understand how to integrate vector databases with AI models effectively and how these technologies fit into the broader AI and data infrastructure. Provide access to specialized training programs, workshops, or certifications that focus on vector database implementation and optimization.
5. Create a Comprehensive Implementation Plan: Develop a detailed plan that outlines how vector databases will support your AI initiatives across departments and use cases. Ensure this plan is aligned with your broader business goals and incorporates both short-term wins and long-term growth opportunities. Include a timeline for scaling from initial proof of concept to broader deployment.
6. Identify and Mitigate Potential Challenges: Consider challenges such as integration complexity, data migration issues, and potential bottlenecks in data processing. Develop mitigation strategies, such as phased integration, data quality assessments, and performance testing, to address these challenges proactively.
7. Collaborate with Experts: Consider partnering with AI experts or cloud service providers with a track record of successfully implementing vector databases for large-scale AI projects. Their experience can help you navigate common challenges, avoid pitfalls, and accelerate your progress, ensuring a smoother transition.
8. Post-Implementation Review: After implementation, conduct a thorough review to assess whether the project met its objectives. Analyze performance data, gather feedback from stakeholders, and identify areas for further optimization. Use these insights to guide future AI initiatives and refine your use of vector databases.
Real-world impact: a financial services example
A global financial services firm recently updated its investment strategy division with vector database technology. By combining vector databases with their existing AI models, they achieved notable improvements:
- They cut the time spent on market research by 40%.
- The accuracy of their investment recommendations increased by 25%.
- They gained the ability to analyze unstructured data from social media and news in real time.
This change went beyond just updating technology—it fundamentally altered how the firm approached data-driven decision-making. The new system allowed them to tap into vast amounts of unstructured data, providing insights that were previously inaccessible or too time-consuming to extract.
Looking forward
As generative AI continues to develop, vector databases will become increasingly important. They’re not just tools for managing data; they’re the foundation for the next wave of AI-powered businesses.
Company leaders who recognize this now and take action will be well-positioned to lead in an AI-driven future. Those who delay may find themselves struggling to catch up in a market where advanced AI capabilities become standard rather than exceptional.
The key question for business leaders isn’t whether to adopt vector databases but how quickly they can integrate them into their AI strategy. In the fast-paced world of generative AI, having the right data infrastructure isn’t just helpful—it’s essential for staying competitive. By implementing vector databases now, you’re not just preparing for the future of AI; you’re actively shaping it to your advantage.
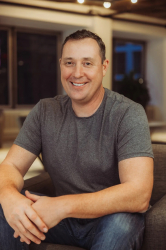