For example, I’ve been playing around with an experimental system I built for myself using GPT-3 designed to help me write a science fiction book, which is something that I’ve wanted to do since I was a teenager. I have notebooks full of synopses I’ve created for theoretical books, describing what the books are about and the universes where they take place. With this experimental tool, I have been able to get the logjam broken. When I wrote a book the old-fashioned way, if I got 2,000 words out of a day, I’d feel really good about myself. With this tool, I’ve had days where I can write 6,000 words in a day, which for me feels like a lot. It feels like a qualitatively more energizing process than what I was doing before.
This is the “copilot for everything” dream—that you would have a copilot that could sit alongside you as you’re doing any kind of cognitive work, helping you not just get more done, but also enhancing your creativity in new and exciting ways.
This increase in productivity is clearly a boost to your satisfaction. Why do these tools bring more joy to work?
All of us use tools to do our work. Some of us really enjoy acquiring the tools and mastering them and figuring out how to deploy them in a super effective way to do the thing that we’re trying to do. I think that is part of what’s going on here. In many cases, people now have new and interesting and fundamentally more effective tools than they’ve had before. We did a study that found using no-code or low-code tools led to more than an 80% positive impact on work satisfaction, overall workload and morale by users. Especially for tools that are in their relatively early stages, that’s just a huge benefit to see.
For some workers, it’s literally enhancing that core flow that you get into when you’re doing the work; it speeds you up. It’s like having a better set of running shoes to go run a race or marathon. This is exactly what we’re seeing with the experiences developers are having with Copilot; they are reporting that Copilot helps them stay in the flow and keeps their minds sharper during what used to be boring and repetitive tasks. And when AI tools can help to eliminate drudgery from a job, something that is super repetitive or annoying or that was getting in their way of getting to the thing that they really enjoy, it unsurprisingly improves satisfaction.
Personally, these tools let me be in flow state longer than I was before. The enemy of creative flow is distraction and getting stuck. I get to a point where I don’t know quite how to solve the next thing, or the next thing is, like, “I’ve got to go look this thing up. I’ve got to context switch out of what I was doing to go solve the subproblem.” These tools increasingly solve the subproblem for me so that I stay in the flow.
In addition to GitHub Copilot and DALL∙E 2, AI is showing up in Microsoft products and services in other ways. How is next-generation AI improving current products such as Teams and Word?
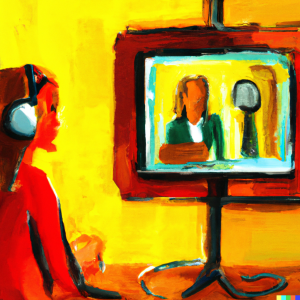
This is the big untold story of AI. To date, most of AI’s benefits are spread across 1,000 different things where you may not even fully appreciate how much of the product experience that you’re getting is coming from a machine learned system.
For example, we’re sitting here in this Teams call on video and, in the system, there are all these parameters that were learned by a machine learning algorithm. There are jitter buffers for the audio system to smooth out the communication. The blur behind you on your screen is a machine learning algorithm at work. There are more than a dozen machine learning systems that make this experience more delightful for the both of us. And that is certainly true across Microsoft.
We’ve gone from machine learning in a few places to literally 1,000 machine learning things spread across different products, everything from how your Outlook email client works, your predictive text in Word, your Bing search experience, to what your feed looks like in Xbox Cloud Gaming and LinkedIn. There’s AI all over the place making these products better.
One of the big things that has changed in the past two years is it used to be the case that you would have a model that was specialized to each one of these tasks that we have across all our products. Now you have a single model that gets used in lots of places because they’re broadly useful. Being able to invest in these models that become more powerful with scale—and then having all the things built on top of the model benefit simultaneously from improvements that you’re making—is tremendous.
Microsoft’s AI research and development continues through initiatives such as AI4Science and AI for Good. What excites you most about this area of AI?
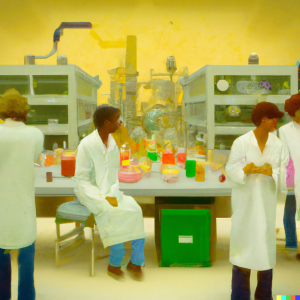
The most challenging problems we face as a society right now are in the sciences. How do you cure these intractably complicated diseases? How do you prepare yourself for the next pandemic? How do you provide affordable, high-quality healthcare to an aging population? How do you help educate more kids at scale in the skills that they will need for the future? How do you develop technologies that will reverse some of the negative effects of carbon emissions into the atmosphere? We’re exploring how to take some of these exciting developments in AI to those problems.
The models in these basic science applications have the same scaling properties as large language models. You build a model, you get it into some self-supervised mode where it’s learning from a simulation or it’s learning from its own ability to observe a particular domain, and then the model that you get out of it lets you dramatically change the performance of an application—whether you’re doing a computational fluid dynamics simulation or you’re doing molecular dynamics for drug design.
There’s immense opportunity there. This means better medicines, it means maybe we can find the catalyst we don’t have yet to fix our carbon emission problem, it means across the board accelerating how scientists and other folks with big ideas can work to try to solve society’s biggest challenges.
How have breakthroughs in computing techniques and hardware contributed to the advances in AI?
The fundamental thing underlying almost all of the recent progress we’ve seen in AI is how critical the importance of scale has proven to be. It turns out that models trained on more data with more compute power just have a much richer and more generalized set of capabilities. If we want to keep driving this progress further—and to be clear, right now we don’t see any end to the benefits of increased scale—we need to optimize and scale up our compute power as much as we possibly can.
We announced our first Azure AI supercomputer two years ago, and at our Build developer conference this year I shared that we now have multiple supercomputing systems that we’re pretty sure are the largest and most powerful AI supercomputers in the world today. We and OpenAI use this infrastructure to train nearly all of our state-of-the-art large models, whether that’s our Turing, Z-code and Florence models at Microsoft or the GPT, DALL∙E and Codex models at OpenAI. And we just recently announced a collaboration with NVIDIA to build a supercomputer powered by Azure infrastructure combined with NVIDIA GPUs.
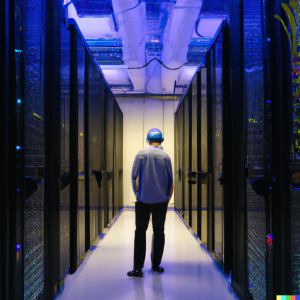
Some of this progress has just been via brute force compute scale with bigger and bigger clusters of GPUs. But maybe even a bigger breakthrough is the layer of software that optimizes how models and data are distributed across these giant systems, both to train the models and then to serve them to customers. If we’re going to put forth these large models as platforms that people can create with, they can’t only be accessible to the tiny number of tech companies in the world with enough resources to build giant supercomputers.
So, we’ve invested a ton in software like DeepSpeed to boost training efficiency, and the ONNX Runtime for inference. They optimize for cost and latency and generally help us make bigger AI models more accessible and valuable for people. I’m super proud of the teams we have working on these technologies because Microsoft is really leading the industry here, and we’re open sourcing all of it so others can keep improving.
These advances are all playing out amid an ongoing concern that AI is going to impact jobs. How do you think about the issue of AI and jobs?
We live in a time of extraordinary complexity and historic macroeconomic change, and as we look out 5, 10 years into the future, even to just achieve a net neutral balance for the whole world, we’re going to need new forms of productivity for all of us to be able to continue enjoying progress. We want to be building these AI tools as platforms that lots of people can use to build businesses and solve problems. We believe that these platforms democratize access to AI to far more people. With them, you’ll get a richer set of problems solved and you’ll have a more diverse group of people being able to participate in the creation of technology.
With the previous instantiation of AI, you needed a huge amount of expertise just to get started. Now you can call Azure Cognitive Services, you can call the Azure OpenAI Service and build complicated products on top of these things without necessarily having to be so expert at AI that you’ve got to be able to train your own large model from scratch.