In this interview, Dr. Jo Varshney, Co-Founder and CEO of VeriSIM Life, sheds light on the groundbreaking potential of AI-driven biosimulation in transforming drug development. VeriSIM Life’s mission is to accelerate the drug discovery process by eliminating the inefficiencies of traditional methods, particularly animal testing.
By leveraging advanced machine learning models, their platform accurately predicts drug efficacy and safety in humans, drastically reducing the time and cost of bringing new treatments to market. Dr. Varshney also discusses the ethical implications of using biosimulation as an alternative to animal testing, the challenges of gaining industry acceptance, and how their technology is being integrated into pharmaceutical pipelines. With AI rapidly advancing, VeriSIM Life is poised to play a significant role in the future of healthcare and beyond.
1. Can you explain the core mission of VeriSIM Life and how your AI-driven biosimulation technology is transforming the drug development process?
Our mission at VeriSIM Life is to eliminate inaccuracy and waste when translating drug candidates to clinical trials using AI-augmented, multi-disciplinary quantitative methods that predict patient outcomes.
We believe that the current approach to drug discovery and development is unsustainable. The cost and time it takes to bring drugs to market has doubled every 10 years. The pharma industry spends an estimated $300 billion on R&D a year, while the FDA approves only about 50 new drugs. Meanwhile, 300 million patients with unmet diseases continue to await therapies.
We aim to change this paradigm by using deep technology to unwind biology. Our technology predicts which drug candidates are most likely to succeed in clinical trials before they enter the trials, to reduce trial and error in R&D, and get new drugs to patients faster.
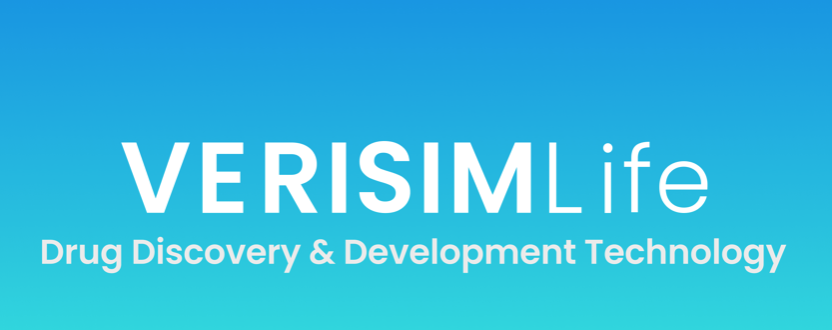
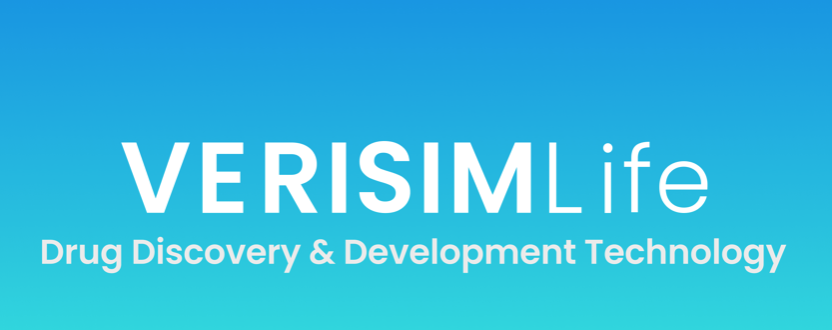
2. What inspired you to focus on alternatives to animal testing, and how does biosimulation provide a more ethical and effective solution?
My parents were involved with the biopharmaceutical industry, so I was exposed to and developed an interest in science, technology, and drug development from an early age. I saw first-hand the role of animal testing in the drug discovery process and noticed that it actually has limited value for predicting human outcomes, specifically drug safety and efficacy. I started thinking more about the drug R&D process to explore if animal testing was truly essential to the extent it has been for so many years.
After studying comparative oncology, genomics and bioinformatics, I realized more acutely how difficult it is to translate from the lab to clinical trials and it got me thinking, there must be a better, efficient way to help identify clinical risks and avoid or reduce the errors. So, I studied computer science to use machine learning, mathematical models, and data to see how a new drug might work in humans. I coded a virtual mouse and simulated its reaction to a drug with publicly available data and compared the output for matches. It was incredibly accurate and actually won a Google-sponsored innovation challenge.
That was what kick-started VeriSIM Life. And now our technology can predict drug efficacy and safety with an average of 83% accuracy (often well over 90%) across various animal species and humans. By using AI aided computer simulations, we can reduce unnecessary animal experiments while improving the success rate of human trials.
3. How does your technology compare to traditional animal testing methods in terms of accuracy, speed, and cost-effectiveness?
Our platform is actually more accurate than animal models in predicting human drug responses because it can be specifically designed to analyze human-specific data, addressing the inherent limitations posed by differences for example in enzymes, metabolic pathways, and overall physiology between animals and humans. These biological differences lead to discrepancies between how drugs behave in animal models versus in human trials. This misalignment contributes to the high failure rates seen in drug development and raises ethical concerns about animal treatment.
But beyond the ethical concerns, new classes of medicines introduce additional scientific and practical challenges. These complex therapeutics often interact with human biological systems in ways that are not accurately replicated in animal models due to species-specific differences. For example, the immune system of animals living in controlled environment can react very differently from that of humans, leading to misleading data on safety and efficacy.
AI can address these challenges by leveraging large datasets from human biology, including genomics, proteomics, and clinical data, to create more accurate and predictive models. These AI-driven models can simulate human biological processes computationally, providing rapid insights that are more relevant to human health and disease. Additionally, AI can integrate and analyze complex datasets that would be difficult to interpret using traditional methods, leading to more informed decision-making in drug development. This approach is also extremely more cost-effective than animal testing.
4. Could you share some specific examples where your biosimulation platform has successfully predicted drug efficacy or toxicity, potentially avoiding the need for animal testing?
Recently, one of our pharmaceutical partners, Debiopharm, asked us to help them with the development of antibody-drug conjugates (ADCs) for treating acute myeloid leukemia (AML) and diffuse large B-cell lymphoma (DLBCL). By utilizing our hybrid-AI approach, we were able to simulate the efficacy and synergy of drug combinations computationally, which allowed them to focus on the most promising candidates. This approach not only reduced the number of required animal studies but also optimized the drug development process by identifying the most effective therapies early on. In this specific case, the use of our Translational Index further guided decision-making, ensuring that only the highest-probability candidates advanced to in vivo studies, thus minimizing unnecessary animal testing.
5. What challenges have you faced in gaining industry acceptance for AI-driven alternatives to animal testing, and how have you overcome them?
In an industry built on the scientific method, AI-driven approaches have always been viewed with skepticism. The biggest objection traditional scientists have with AI is the lack of explainability, or the “black box” phenomenon. On top of that, you have the real issue of bias skewing the veracity of AI-derived insights, especially when working from limited datasets.
We’ve been thinking a lot about explainable AI, which is one of the reasons that our approach is different. We combine AI with mechanism-based systems to provide explainability into our results. Those results are expressed in a metric we call Translational Index™–akin to credit score. Translational Index provides transparent, interpretable insights into our models’ decision-making processes. This analysis allows us to understand the importance of molecular “features” that contribute to each clinical characteristic. It also identifies the complex interaction effects between different criteria.
6. How does VeriSIM Life’s technology integrate with existing drug development pipelines, and what are the implications for pharmaceutical companies?
We collaborate with clients in a number of ways. For existing drug development pipelines, we deliver BIOiSIM-enabled professional services to address an asset’s specific translational challenges, and achieve more successful clinical trial outcomes.
For clients earlier in the discovery process, we partner with biotech and pharma clients to identify successful novel candidates for difficult targets. Our AtlasGEN Novel Drug Designer has the unique ability to merge biological relevance with target engagement chemistry, designing-in clinical success from day one. This reduces investigation of thousands of potentially dead-end compound “hits” to a handful of promising drug candidate leads.
7. What role does regulatory approval play in the adoption of AI-driven biosimulation as a standard practice, and how are you engaging with regulatory bodies to advance this cause?
Regulatory agencies like the FDA are becoming increasingly receptive to alternative approaches, including AI-driven methods. The FDA’s Innovative Science and Technology Approaches for New Drugs (ISTAND) Pilot Program now welcomes submissions for qualifying drug development tools such as AI. In collaboration with regulators, we are co-leading an AI initiative with FDA experts to accelerate the adoption and qualification of AI-driven methodologies, aiming to reduce reliance on traditional animal studies while maintaining the highest standards of safety and efficacy in drug development.
8. Looking to the future, how do you see the landscape of drug development evolving with the increasing reliance on AI and machine learning technologies?
We’re still waiting to see how deeply AI will be woven into the drug development lifecycle. A lot of early focus was on the discovery phase–identifying disease targets and the best potential compounds to engage those targets. Another wave of applications was focused on the clinical trial phase–helping companies improve the design, recruiting and management of trials. Honestly, we are the only company I’m aware of that is primarily focused on the preclinical translation phases. I see a lot more evolution in this aspect of drug development. All the investment into AI across the industry is good news for patients. It will eventually result in more treatment options and lower costs.
9. Beyond drug development, do you see potential applications for biosimulation technology in other areas of healthcare or scientific research?
Biosimulation technology holds significant potential beyond drug development, particularly in areas such as repurposing or redirecting drug assets. By leveraging advanced modeling and simulation, we can explore new therapeutic applications for existing drugs, potentially saving years in development and reducing costs. This approach enables more efficient drug repositioning, especially for diseases with unmet needs, while also providing a faster route to market for innovative treatments.
In addition, biosimulation can play a transformative role in agriculture by enhancing crop resilience and optimizing the use of pesticides and fertilizers, improving food security. Moreover, it can be used to identify biological threats, such as pathogens or emerging diseases, and help design proactive strategies to combat these threats. This application could revolutionize preparedness and response efforts in both public health and environmental sectors, improving overall societal resilience to future biological challenges.
10. What advice would you give to other innovators looking to disrupt traditional practices in scientific research with AI and other emerging technologies
My advice is to embrace the resistance that many in the scientific community will put in front of you. Keep working on the big problems and making progress. We’re finally seeing that resistance start to weaken, but it’s pretty pervasive. For women especially, making in-roads with innovation into traditional STEM-related fields hasn’t been easy. If you’re a female founder, don’t get discouraged. Keep fighting for your mission, and surround yourself with a team that believes equally in your vision.