October 22, 2024
4 min read
By Cogito Tech.
92 views
Until recently, large language models (LLMs) were primarily trained on English and a few other widely spoken languages, limiting their applicability to less common languages and cultural contexts. To address this concern, OpenAI recently released Multilingual Massive Multitask Language Understanding (MMMLU) datasets to expand the global reach of artificial intelligence. This dataset can be used to test the ability of language models to understand and respond in 14 languages, including Arabic, Bengali, and German. This step is helpful for enterprises whose AI solutions face barriers entering emerging markets due to language limitations.
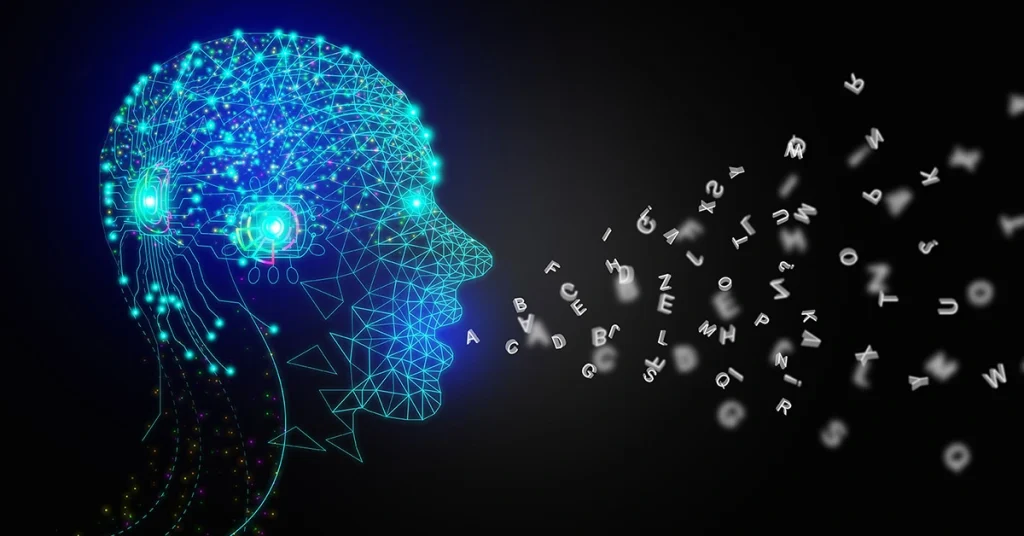
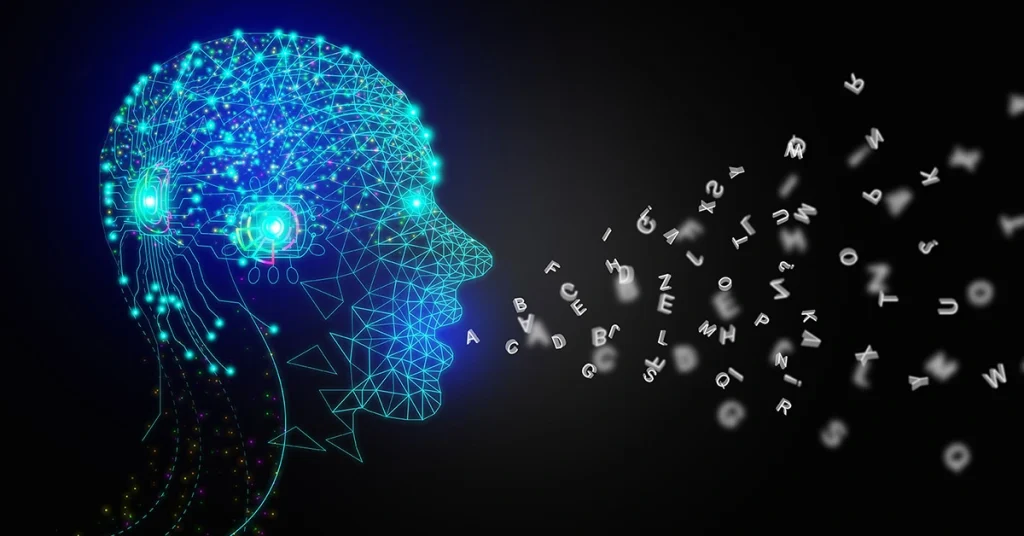
Multilingual AI data is essential to make AI technologies, particularly Natural Language Processing (NLP) models, more accessible and inclusive for all, irrespective of the language people speak. Developing AI algorithms that can process and understand multiple languages is the first step for AI systems to deliver human-like experiences across languages and geographies.
This article explores the importance and use cases of multilingual datasets for LLMs.
Why Multilingual Data is the New Frontier in AI Development
Here are key reasons why multilingual data is important for building AI models.
Cross-Language Connectivity
Even in today’s interconnected world, people communicate in different languages, leaving a significant amount of data and information isolated and inaccessible due to language barriers. This limits the potential value that businesses and users could derive from this information.
Training machine learning models using multilingual data helps to reduce language barriers. Multilingual AI models promote effective communication and interaction across languages, facilitating global collaboration, fostering understanding, and offering businesses a competitive advantage.
Expanding Business Opportunities
Multilingualism is essential in today’s globalized world, enabling businesses to expand their reach to cater to diverse customer bases by understanding and communicating in multiple languages. Similarly, AI models trained on multilingual datasets help businesses extend their services and offerings by providing effective customer service, marketing, and other services in diverse markets.
Bias Mitigation and Cultural Sensitivity
Language is an integral part of culture. Multilingual datasets capture a variety of cultural perspectives and nuances embedded in different languages, helping AI models understand and respond to different contexts appropriately. This diversity enables AI models to generate more culturally sensitive responses and avoid offensive content. Understanding cultural nuances helps AI models offer a better user experience, ultimately leading to improved brand loyalty.
Multi-language Insights Retrieval
While the world stands connected, information is often stored in silos in different languages, making it difficult for people who don’t understand them. The language barrier hampers businesses and users from obtaining valuable insights that can be useful for decision-making.
Training machine language models on diverse, multilingual data converts incomprehensible information into valuable insights. This breaks down language silos, allowing businesses to better understand global trends and markets. This is crucial for driving better decision-making pertaining to specific geographies based on data from various sources, regardless of language.
Research and Development
Multilingual text data helps researchers and developers working on machine learning and natural language processing projects. They create and refine algorithms that process and analyze text in various languages. As a result, the algorithms become more accurate and effective in tasks such as translation, sentiment analysis, and text summarization.
Multilingual AI Data Use Cases
Here are a few top use cases of multilingual AI data:
Sentiment Analysis: Multilingual data helps to analyze sentiment in customer feedback, reviews, and social media across languages. Businesses that operate across geographies can use multilingual sentiment analysis to understand customer feedback and adapt operational strategies accordingly.
Machine Translation: It plays a critical role in translating text from one language to another. For example, Google Translate makes translation from one language to another using multilingual AI text data.
Content Localization: Streaming services can use multilingual AI to adapt and translate content for different geographies, generating subtitles and content recommendations based on users’ language preferences.
Content Moderation: Multilingual AI models can be used to moderate and filter user-generated content across languages on social media to identify and remove inappropriate content in different languages.
Cross-language Information Retrieval: Multilingual AI bots help retrieve valuable information from documents written in different languages. For example, search engines powered by multilingual AI provide users with search results in their preferred language, even when they query in other languages.
Customer Support: AI chatbots can simplify customer interactions by providing customer support to resolve their queries and provide information in their preferred language.
Extracting Insights from Legal Documents: Legal firms can employ such AI models to quickly process and analyze legal documents and contracts written in different languages to identify potential legal issues or risks. It assists lawyers who are not fluent in a particular language by translating or providing summaries of legal documents.
Market Trends and Competition Analysis: Multilingual AI can analyze text data from various sources in different languages to gain valuable insights into market trends, competitor strategies, and consumer behavior across geographies. This helps businesses make informed decisions about product development, marketing, and business expansion.
Language-agnostic Messaging App: Multilingual AI-powered applications allow users to communicate in their preferred language seamlessly. This feature eliminates the need for users to rely on translation tools or switch languages, which is particularly useful for businesses serving diverse customer bases.
Challenges of Multilingual AI Datasets
While multilingual AI models can expand business opportunities across demographics, models must be fed large volumes of multilingual and grammatically, contextually, and factually correct training datasets.
However, sourcing quality multilingual AI text data poses huge challenges for industries, necessitating an extra layer of validation to ensure accuracy and appropriateness. The process also involves linguists and language experts to curate multilingual datasets for effective polyglot AI successfully.
Cogito’s Expertise in Multilingual AI Data Labeling and Curation
With nearly a decade of experience in text data labeling and curation, Cogito specializes in delivering high-quality multilingual AI training data that fuels innovative machine learning solutions. We leverage an expert-in-the-loop approach to carefully examine and validate input data, implementing effective annotation procedures. Our globally distributed multilingual workforce comprises fluent native speakers with specialized language expertise. Our team also excels in complex, multilingual writing and text translation tasks.
Moreover, we can scale our operations to meet any volume, format, or industry-specific requirements. Leveraging our expertise, our team efficiently sources, compiles, labels, and curates high-quality text and audio data in the precise language you require.