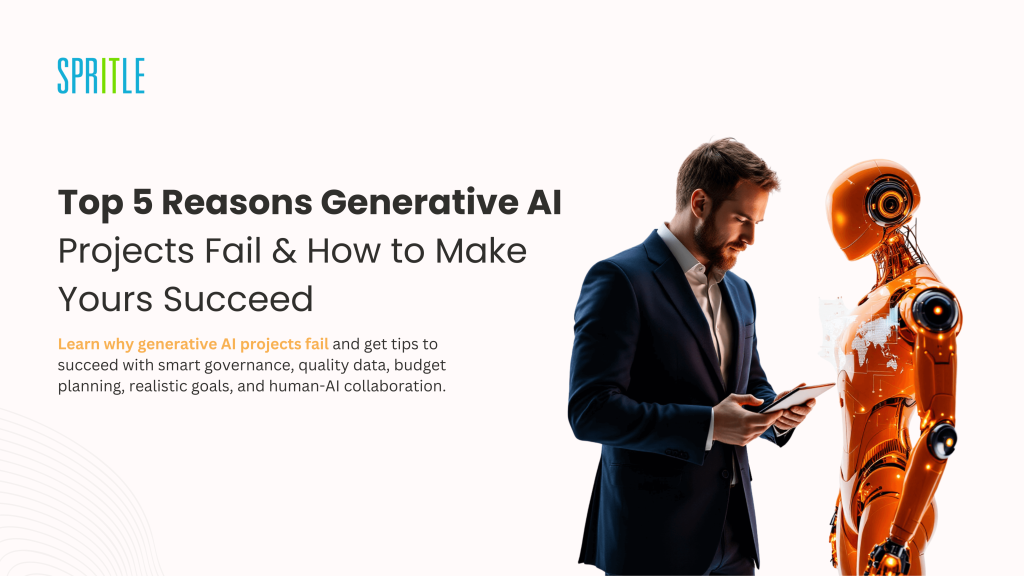
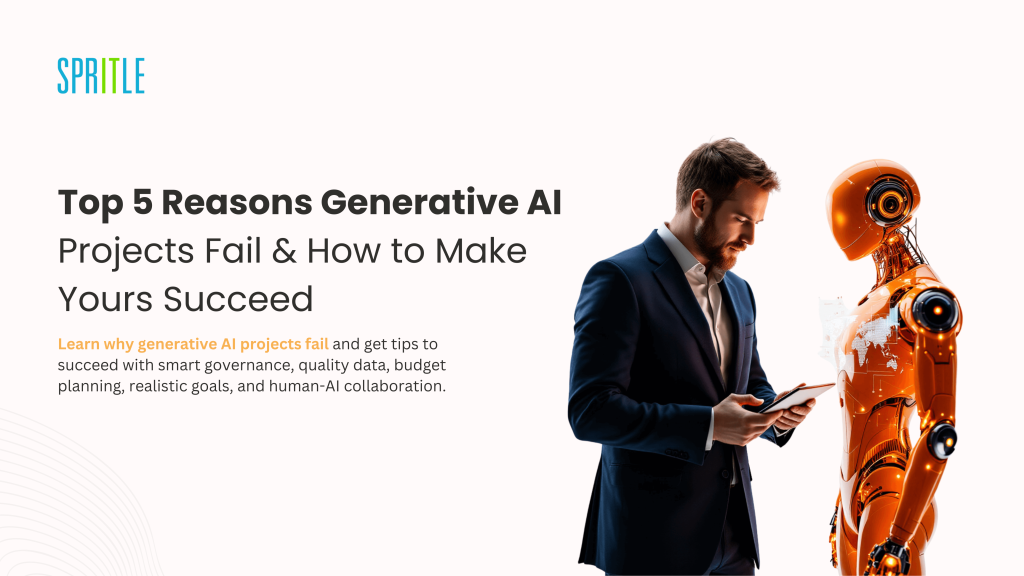
Welcome, AI Enthusiasts! 🤝
Generative AI is reshaping industries from retail to legal and supply chain management. However, many generative AI projects fall short due to specific challenges that, if addressed, can pave the way to greater success. This blog examines five primary reasons for these failures and offers actionable strategies. Real-world examples and industry data illustrate these pitfalls, providing a roadmap for successful AI implementation.
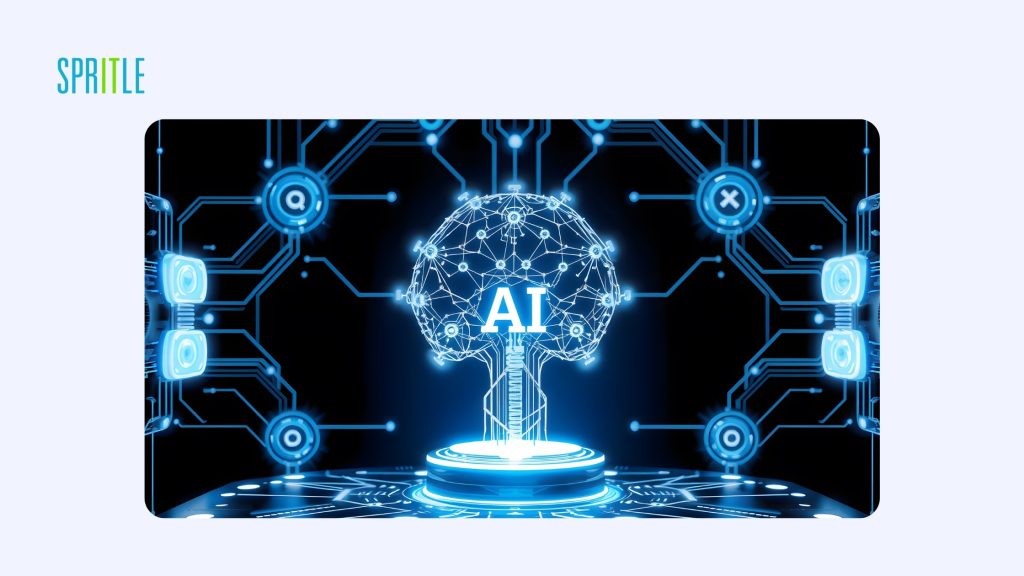
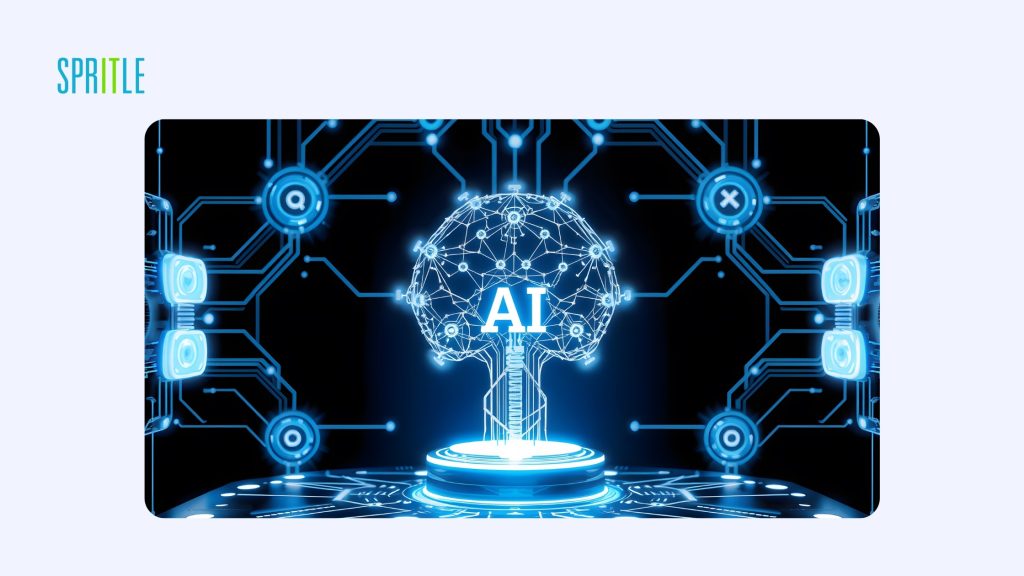
Why Generative AI Projects Fail : 5 Reasons and How to Succeed
Learn the top five reasons why generative AI projects frequently fail and gain insights to help your project succeed. This guide highlights common challenges, including data constraints, model alignment, and scaling issues, offering practical solutions to overcome each.
Top 5 Reasons Generative AI Projects Fail
Whether you’re beginning a new AI venture or enhancing an existing one, these tips will equip you to navigate obstacles and drive impactful results with generative AI.
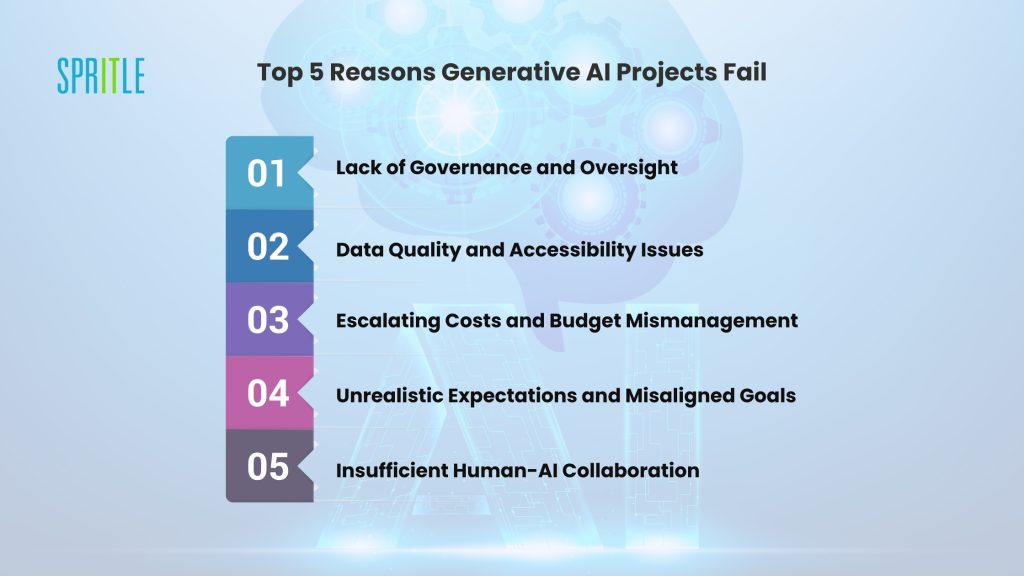
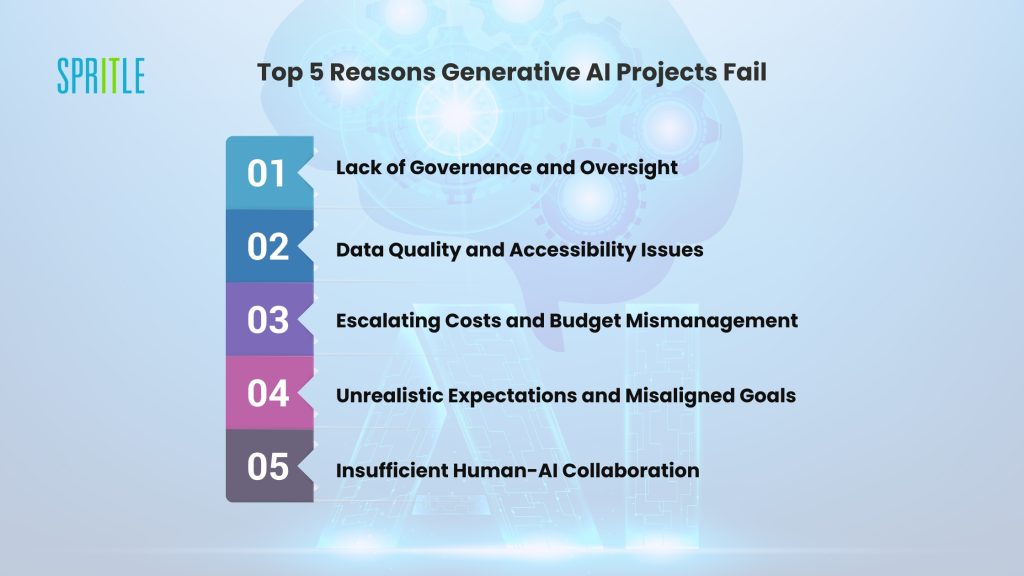
1. Lack of Governance and Oversight
Why It Fails:
Governance is essential for generative AI projects. Without robust oversight, projects can produce biased, harmful, or inaccurate outputs, potentially leading to reputational and financial damage.
- According to Gartner, by 2025, over 80% of AI projects are expected to generate incorrect or biased results due to poor oversight and governance structures. Additionally, 42% of companies report experiencing at least one “significant” AI-related ethical issue since launching their AI systems.
Case in Point: Pak’nSave’s Savey Meal-Bot
Pak’nSave, a New Zealand-based supermarket, introduced a bot allowing customers to input ingredients and receive recipe suggestions. However, a lack of governance led to incidents where the bot suggested recipes with toxic substances, like bleach. The bot’s unregulated output attracted global media attention, emphasizing the risks of deploying AI without adequate oversight.
Strategies to Overcome This:
- Incorporate Ethical Guidelines: Set clear ethical boundaries to prevent dangerous suggestions, like those made by Pak’nSave’s bot.
- Establish Compliance and Accountability: Include legal compliance and define clear responsibility across developers, data scientists, and managers.
- Implement Monitoring and Human Oversight: Regular quality assurance and human-in-the-loop models can catch errors early, preventing issues before they escalate.
Solutions at a Glance:
Build a governance framework including ethical guidelines, accountability structures, and real-time monitoring. Integrate human oversight and feedback mechanisms to ensure that AI aligns with safety, ethical, and legal standards.
2. Data Quality and Accessibility Issues
Why It Fails:
Generative AI relies heavily on data, making data quality and accessibility paramount. Poor-quality or inaccessible data leads to inaccurate outputs, while data silos within organizations can prevent cohesive datasets, hindering AI’s performance.
- A recent survey by VentureBeat found that 87% of data science projects never make it to production, with data quality issues being a top reason. Moreover, McKinsey estimates that poor data quality costs the U.S. economy approximately $3.1 trillion annually.
Case in Point: Supply Chain AI at a Chip Manufacturer
A chip manufacturer attempted to optimize its supply chain using AI but struggled due to fragmented data across departments. This lack of standardized data delayed insights and limited the AI’s potential effectiveness.
Strategies to Overcome This:
- Centralize and Standardize Data: Breaking down data silos and standardizing data across departments can increase AI’s accuracy.
- Ensure Access to Real-Time Data: Outdated or incomplete data can lead to poor insights; access to real-time, updated data is crucial.
- Maintain Data Privacy and Security Standards: With 76% of consumers concerned about data privacy, ensuring secure data handling is essential to avoid reputational and financial risks.
Solutions at a Glance:
Centralize and standardize data, ensure real-time access, and establish data-cleaning protocols. Use well-labeled data and secure sources to enhance AI’s accuracy and reliability.
3. Escalating Costs and Budget Mismanagement
Why It Fails:
Generative AI is often considered cost-effective at first, but expenses can quickly increase as projects scale. From data storage to API usage, scaling without budget foresight can make AI projects financially unsustainable.
- According to IDC, 70% of AI projects experience cost overruns, often due to underestimated storage and processing needs. Additionally, the average cost of training a large language model can exceed $1 million, with some projects running much higher due to ongoing optimization and tuning costs.
Case in Point: Cost Overruns at a Global Electronics Company
A global electronics company underestimated the costs of AI for large-scale document creation. While initial expenses were manageable, API usage, data storage, and processing demands quickly escalated.
Strategies to Overcome This:
- Forecast Storage and Processing Costs: Predict costs as data requirements grow and plan accordingly.
- Budget for Continuous Model Optimization: Generative AI models require regular updates to stay accurate, so planning for these costs is essential.
- Optimize API Usage: Each API call has a cost, which can multiply quickly at scale; optimizing usage can significantly control expenses.
Solutions at a Glance:
Conduct detailed cost forecasting, allocate funds for data security and compliance, and monitor API usage. Build flexibility into budgets to cover ongoing model optimization and unanticipated costs.
4. Unrealistic Expectations and Misaligned Goals
Why It Fails:
Generative AI is powerful but isn’t a one-size-fits-all solution. Unrealistic expectations and misaligned goals can lead to disappointment, project failure, or abandonment.
- In a 2023 study by Deloitte, 63% of executives said their AI projects fell short of expectations due to misaligned goals. Additionally, a recent survey found that 55% of organizations admitted they lack clearly defined AI success metrics, making it difficult to gauge project performance effectively.
Case in Point: Document Creation at a US Electronics Manufacturer
An electronics company attempted to use AI to create customized pricing documents. They expected the AI to autonomously generate accurate pricing, which it couldn’t fulfill without human input. Misaligned expectations led to frustration and delays.
Strategies to Overcome This:
- Educate Stakeholders on AI Capabilities: Help stakeholders understand AI’s strengths and limitations to prevent over-promising.
- Set Clear Success Metrics: Define performance metrics to evaluate the AI’s success meaningfully.
- Differentiate Between Short-Term and Long-Term Goals: Outline both short- and long-term goals to ensure the project delivers sustainable value.
Solutions at a Glance:
Set realistic expectations with well-defined success metrics, align projects with strategic goals, and communicate effectively with stakeholders. Properly plan for both short-term and long-term resource needs.
5. Insufficient Human-AI Collaboration
Why It Fails:
Generative AI excels at automating tasks but lacks the nuanced judgment required for many applications. Without human oversight, AI can produce outputs that are insensitive, inaccurate, or potentially harmful.
- According to a 2023 survey by McKinsey, 55% of organizations reported that AI failures were directly linked to inadequate human oversight. Additionally, 58% of executives highlighted that integrating human review processes significantly improved the accuracy and quality of AI outputs.
Case in Point: Legal Document Errors at Levidow, Levidow & Oberman
Law firm Levidow, Levidow & Oberman used ChatGPT to draft legal documents, which included fabricated citations. This reliance on AI without human review led to reputational damage and fines, highlighting the importance of human oversight.
Strategies to Overcome This:
- Prioritize Feedback Loops: Regular human feedback is essential to continuously refine AI models.
- Provide Role-Specific AI Training: Departments using AI should have training tailored to their needs, maximizing AI’s potential.
- Combine Human and AI Decision-Making: Use AI to support decision-making rather than replace it, ensuring high-quality outcomes.
Solutions at a Glance:
Combine human and AI expertise with feedback loops, cross-functional collaboration, and role-specific training. Allocate resources for human oversight and ensure ethical checks are in place to achieve optimal results.
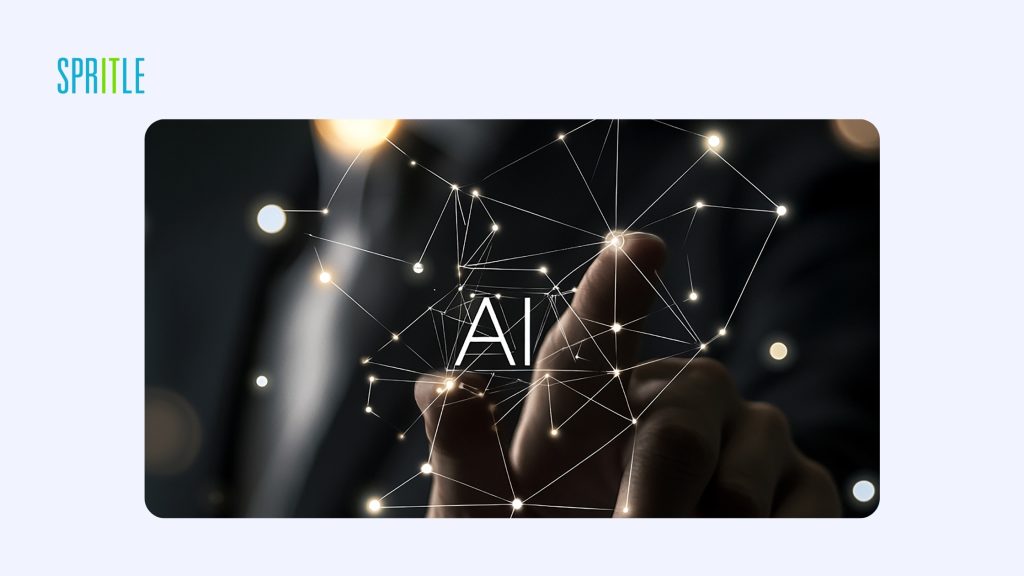
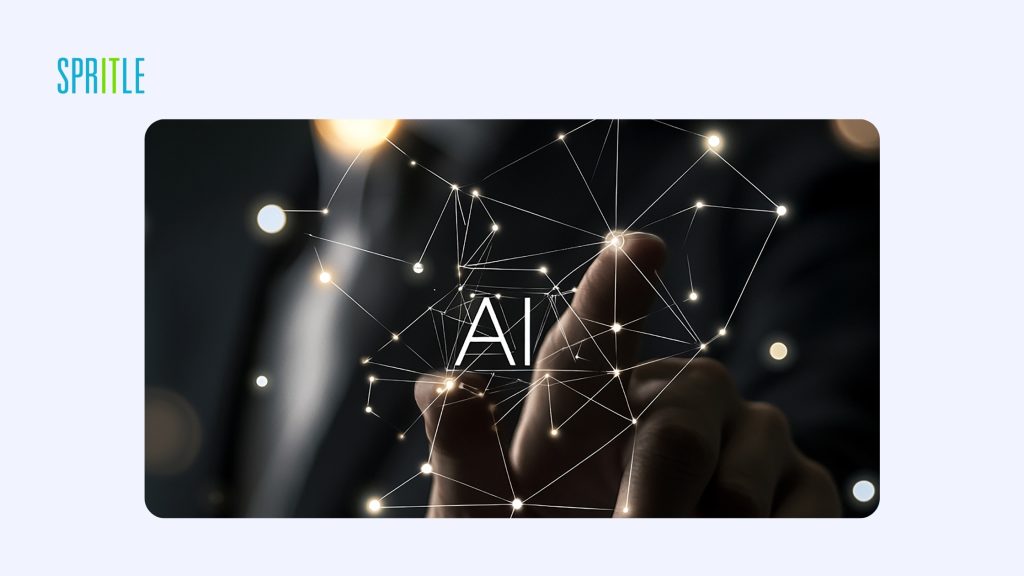
Pro Tip :
Continuous Adaptation in the AI Landscape
Generative AI is rapidly evolving, making adaptability a key success factor. Keeping up with new tools, updating models, and monitoring compliance ensures projects remain effective and relevant.
Strategies for Continuous Adaptation:
- Regular Model Updates: Retraining models helps counteract emerging biases. A study by IBM found that organizations that updated AI models quarterly saw a 25% improvement in output quality.
- Adopt New Techniques: Staying current with AI advancements boosts project performance.
- Prioritize Compliance: With over 75 new AI regulations introduced in 2023 alone, staying compliant helps organizations avoid legal repercussions.
Final Thoughts
Generative AI has the potential to revolutionize industries, but success requires clear governance, high-quality data, realistic goals, human collaboration, and adaptability. By addressing these five key areas with additional layers of oversight, structure, and adaptability, organizations can reduce the risk of failure and fully leverage AI’s transformative power.
Are you ready to unlock the potential of generative AI? Start by building a strong foundation with well-defined goals, resource planning, and a collaborative approach that ensures generative AI projects deliver value and align with organizational priorities.